Statisticians and Wildfires at the Wildland/Urban Interface
Christopher K. Wikle is Curators’ Distinguished Professor of Statistics at the University of Missouri with additional appointments in soil, environmental, and atmospheric sciences and the Truman School of Public Affairs. His primary research interests are in spatiotemporal statistics applied to environmental and ecological processes, with particular interest in dynamics. He is a fellow of the American Statistical Association, Institute of Mathematical Statistics, and International Statistical Institute and has received the ASA Statistics and the Environment Section Distinguished Achievement Award.
Jonathan R. Bradley is an associate professor in the Florida State University Department of Statistics. His primary interests include Bayesian analysis and spatiotemporal statistics with applications to environmental processes and official statistics.
Millions of acres of land are destroyed by wildfires every year, and they pose a significant threat to humans in terms of property damage and potential loss of life. Of course, there is a significant effect on the ecology of areas affected by wildfires, as well.
The risk of devastating fires and their costs have increased due to trends in land development near the boundary between wildland and urban areas, which is known as the wildland urban interface (WUI). Additionally, there is evidence that wildfires are becoming bigger and more common, and this trend is predicted to continue because of global warming.
This is presenting ever greater challenges to those who must manage, mitigate, and predict fires at the WUI. In addition to the increasing economic burden, the main responsibility during an active wildfire is to protect or evacuate people and mitigate the effects on property and infrastructure. Therefore, it is crucial to develop trustworthy models that can anticipate potential dangers from imminent wildfires to human populations and offer a mechanism for quantifying uncertainty that enables the creation and evaluation of feasible mitigation and prediction strategies.
Although statisticians have been involved in various aspects of wildfire modeling for years, greater numbers of megafires, new data sources, and methodological advancements in statistics and data science are leading to increasing involvement.
Wildfire risk assessment and modeling at the WUI provides a perfect example of the need for engagement from a variety of stakeholders, including federal, state, and local governments and NGOs. Indeed, these various stakeholders often have competing interests and objectives, which leads to substantial disagreement about managing forests and mitigating fire risk.
One consequence of this uncertainty is the development of more systematic and empirical-based assessments of forest resiliency (i.e., the capacity of an ecosystem to rebound or adapt after disturbance). It is becoming clear that this requires an understanding of multiple mechanisms across a wide range of spatial and temporal environmental scales, as well as trophic levels in the ecosystem.
Substantive evaluation of these processes must consider a wide range of data and more sophisticated statistical methods. Fortunately, there are increasingly large amounts of publicly available, georeferenced environmental and ecological data and administrative records available to assist modelers and data analysts in these endeavors.
In addition to forest resiliency, there is still a great need for statisticians to contribute to several fire-specific areas of inquiry. These include predicting fire occurrence risk (e.g., the probability of ignition), fire growth (i.e., rate of spread, size), and fire frequency (e.g., the annual area burned in a region, the interval between fire arrivals on the landscape and burn probability after ignition).
One example of how current advances in statistical methodology are already affecting WUI research is spatial and spatiotemporal extreme value analysis. This is an area, mainly coming out of the environmental statistics community, that is rapidly advancing due to the need to provide greater understanding of the potential for extreme outcomes associated with global and regional climate change. Although the evaluation of wildfire risk has been considered by statisticians for quite some time, the advancements in spatial extremes modeling are providing ever greater understanding of these risks across the landscape. These approaches are now being combined with machine learning models to enhance risk assessment. The topic is of such importance that a wildfire risk data set was featured at the 2021 Extreme Value Analysis conference data competition.
Another major area in wildfire modeling where statisticians are starting to play a bigger role is wildfire spread. Modeling wildfire spread requires an understanding of the pathways in which fires propagate. Generally speaking, there are three ways for a fire to spread: convective heat transfer (where the flame directly contacts a source of suitable fuel); radiant exposure (where heat from nearby large flames ignites suitable fuel); and firebrand shower (or “spotting,” when new ignition occurs far from the current fire). The first two mechanisms spread the fire in a somewhat continuous manner, but firebrand shower spotting leads to new fires away from the primary fire front and has been demonstrated to be the primary factor in significant conflagrations at the WUI that causes rapid spread.
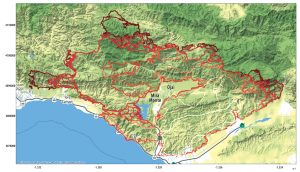
Fire perimeter evolution for the Thomas Fire from December 2017–January 2018 in southern California; lighter lines correspond to earlier times.
The majority of operational wildfire spread models are empirically based, use only a small amount of observational data from past fires, and frequently assume the environment is homogenous and well-known. When flames are burning over homogenous fuel sources with relatively steady winds and level terrain, such models function reasonably well. They do not, however, work well for intense fires under varying settings (e.g., steep terrain, rapidly changing atmospheric conditions, multiple fuel types, extremely dry conditions) or when the fire itself modifies the local weather.
In these situations, models that combine an atmospheric model with a fire-spread component have shown to be substantially more accurate. Unfortunately, these models are expensive and not currently feasible to run in real-time, do not provide uncertainty quantification, and do not adequately account for spotting.
A longstanding approach to parameterize fire fronts for wildfire spread is representing the front as a level set and advecting it according to some empirical-based rate-of-spread formula. These models have recently been cast in a Bayesian state-space setting and used to assimilate data in real time, as well as to include rate of spread parameter estimation and uncertainty quantification. They can also be placed in a Bayesian hierarchical model framework and, with speed of propagation, considered as a Gaussian process. This allows one to predict the propagation of the fire front and characterize its uncertainty.
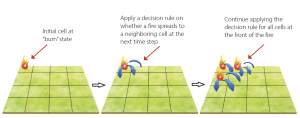
Illustration of a probabilistic cellular automata model for the evolution of a fire on a discrete grid.
Another longstanding approach for modeling wildfire spread is based on cellular automata (CA). CA models dynamically evolve an agent, or automata (i.e., a location referred to as a cell), based on the state (e.g., burning, not burning) of the cell’s “neighbors.” Although these models are used by many subject-matter scientists, the statistical theory of such agent-based models is an important area that needs development where the vast majority of such models have deterministic transition rules based on empirical analysis. These models have been formulated as stochastic models using a rigorous Bayesian hierarchical modeling framework. However, there have only been a few recent examples of fully Bayesian models in the context of wildfire spread prediction. Hence, developing CA models for wildfire spread under a formal Bayesian uncertainty quantification (UQ) framework is an important emerging area.
Recently, CA models have been combined with deep learning methodology without using formal UQ. The development of CA models for wildfires using deep learning methodology is in line with much of the current methodological development in the prediction of wildfires. Recently, deep learning methodology has been effectively used for predicting the spread of wildfires, predicting susceptibility of fires, categorizing fuel types, and developing decision support systems.
Given the changing environment due to climate change, ever-increasing human habitation at the WUI, and associated destruction of life and property that is occurring, there is a greater-than-ever need to understand, predict, and develop mitigation strategies for wildland fires. This will require the involvement of governmental and NGO stakeholders, as well as academics. Statisticians are in a unique position to contribute, given the need to account for a vast array of uncertainties and ever-increasing data volumes.
Further Reading
Banks, D. L., and M. B. 2021. Statistical challenges in agent-based modeling. The American Statistician, 75(3):235-242.
Cisneros, D., Y. Gong, R. Yadav, A. Hazra, and R. Huser. 2021. A combined statistical and machine learning approach for spatial prediction of extreme wildfire frequencies and sizes. arXiv preprint.
Dabrowski, J. J., C. Huston, J. Hilton, S. Mangeon, and P. Kuhnert. 2022. Towards data assimilation in level-set wildfire models using Bayesian filtering. arXiv preprint.
Falk, D. A., P. J. van Mantgem, J. E. Keeley, R. M. Gregg, C. H. Guiterman, A.J. Tepley, … and L. A. Marshall. 2022. Mechanisms of forest resilience. Forest Ecology and Management, 512:120–129.
Hazra, A., B. J. Reich, B. A. Shaby, and A. M. Staicu. 2018. A semiparametric spatiotemporal Bayesian model for the bulk and extremes of the Fosberg Fire Weather Index. arXiv preprint.
Koh, J., F. Pimont, J. L. Dupuy, and T. Opitz. 2021. Spatiotemporal wildfire modeling through point processes with moderate and extreme marks. arXiv preprint.
Li, X., M. Zhang, S. Zhang, J. Liu, S. Sun, T. Hu, and L. Sun. 2022. Simulating forest fire spread with cellular automation driven by a LSTM-based speed model. Fire, 5(1):13.
Taylor, S. W., D. G. Woolford, C. B. Dean, and D. L. Martell. 2013. Wildfire prediction to inform fire management: Statistical science challenges. Statistical Science, 28(4):586–615.
Xi, D. D., S. W. Taylor, D. G. Woolford, and C. B. Dean. 2019. Statistical models of key components of wildfire risk. Annual Review of Statistics and Its Application, 6(1):197–222.
Zhang, L., B. A. Shaby, and J. L. Wadsworth. 2021. Hierarchical transformed scale mixtures for flexible modeling of spatial extremes on datasets with many locations. Journal of the American Statistical Association, 1–13.