Big Data and Better Data
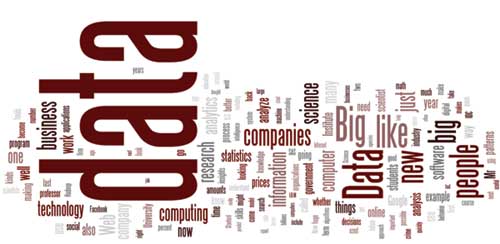
Word cloud created from a dozen recent articles about Big Data. Although statistics is recognized as an important skill, opportunities for the field of statistics are just beginning to unfold.
Big Data is big news. It is the focus of stories in The New York Times and the subject of technology blogs, business forums, and economic studies. This column describes how statisticians can prepare for opportunities in Big Data and explains the distinctive value our profession can provide.
What’s Different About Big Data?
For years, statisticians have been working with large volumes of data in fields as diverse as astronomy, bioinformatics, and data mining. Big Data is different because it is generated on a massive scale by countless online interactions among people, transactions between people and systems, and sensor-enabled machinery.
Big Data is newsworthy because it promises to answer big questions. The potential of Big Data lies in innovative ways it can be linked, related, and integrated to provide more detailed and personalized information than is possible with data from a single source. These innovations make it possible for banks to introduce individually tailored services, for health care providers to offer personalized medicine, and for public safety departments to anticipate crime in targeted areas.
Big Data also is opening doors for researchers and educators. It was the focus of Mathematics Awareness Month, and “Internet scale data” was a topic of Interface 2012. The Statistical and Applied Mathematical Sciences Institute has organized a research program, starting in September, on statistical and computational methodology for massive data sets.
Recently, the Obama administration announced a Big Data research and development initiative, which includes a new solicitation supported by the National Science Foundation (NSF) and National Institutes of Health. NSF also is convening researchers across disciplines to determine how Big Data can transform teaching, and it is encouraging research universities to prepare the next generation of data scientists at all levels.
Are We Data Scientists?
A recurring theme in Big Data stories is the scarcity of “data scientists”—the term used for people who can draw insights from large quantities of data. This shortage was highlighted in an April 26, 2012, Wall Street Journal article titled, “Big Data’s Big Problem: Little Talent”. The question “What is a data scientist?” is still being debated (see the articles with this title at Forbes). However, there is consensus that data scientists must be innovative problemsolvers with expertise in statistical modeling and machine learning, specialized programming skills, and a solid grasp of the problem domain. Hilary Mason, chief data scientist at bitly, adds that “data scientists are responsible for effectively communicating the things that they learn. That might be creating visualizations or telling the story of the question, the answer, and the context.”
Most of these requirements read like the job description for a statistician, but, at a high level, we should view data science as a blend of statistical, mathematical, and computational sciences.
What Do We Need to Learn?
In addition to collaborating with other disciplines on Big Data problems, statisticians must be prepared for a different hardware and software infrastructure. Three developments are noteworthy for us.
First, the scale of terabyte-sized data requires that they be spread across a cluster or grid of multiple computers. Increasingly, the data are held in distributed data stores that are amenable to massively parallel processing, rather than in traditional relational databases.
Second, it is so time consuming to pull distributed data into a computing environment that it has become necessary for computational work to be distributed with the data. Google solved this problem in the context of indexing the web by introducing the MapReduce model for parallel programming. Apache Hadoop, an open-source implementation of this technology, is now widely used for Big Data applications.
Third, the cost of blade servers used in grid systems is dropping. A blade is simply a computer that shares components such as power and cooling to maximize computational ability and minimize space. Commodity blades are cost effective (around $10,000 each), and a rack of 48 blades can provide 1,152 processors, three terabytes of memory, and 20 terabytes of storage. Hundreds or thousands of blades can be added to accommodate more data.
As grid systems become prevalent in data centers and cloud computing services, many statisticians will see greater volumes of data along with rising expectations for analysis. We will need new techniques for data management and new tools for data analysis and visualization. And because so much data come from sources such as mobile phones, social networking sites, and health records, we will also need ways to acquire and analyze unstructured text data.
How Can Big Data Benefit from Us?
While we have much to learn about the domains and technology of large data, the world of Big Data has much to gain from the contributions of statistical scientists. We share many skills with data scientists, but we should proactively explain what sets us apart and why statistical thinking is critical to the process.
Like other analysts, statisticians look for features in large data—and we also guard against false discovery, bias, and confounding. We build statistical models that explain, predict, and forecast—and we question the assumptions behind our models and qualify the use of our models with measures of uncertainty. We work within the limitations of available data—and we design studies and experiments to produce data with the right information content.
If I had to summarize this in a sound bite, I would say that we extract value from data not only by learning from it, but also by understanding its limitations and improving its quality. Better data matters because simply having Big Data does not guarantee reliable answers for Big Questions.
How Should We Respond to Big Data?
Media focus on Big Data could not come at a better time, because the theme for the 2012 Joint Statistical Meetings is “Statistics: Growing to Serve a Data-Dependent Society.” Our presentations should draw attention to statistics as a dynamic discipline that is developing in response to complex, high-dimensional data, as well as new types of data.
We should also take advantage of the spotlight on Big Data to engage students in introductory statistics courses and attract students to statistical careers. And we should actively pursue the opportunities for research, projects, and work force development being created by the administration’s Big Data initiatives.
To keep up with the volume, velocity, and variety of Big Data, we need to stay on top of technological trends and gain new computational skills. This type of training should be offered in our universities and through continuing professional development provided by our association.
The era of Big Data has arrived—and we should think big!
lesson one of word clouds – drop it all to lower case to avoid repetition of key topics. Also, you can probably cut the word data out as it’s obviously going to be there.
Finally, ASA has some voice on Big Data. Thanks to the President of ASA, this is a great article to make statisticians aware of the demand for analyzing and mining Big Data. Although it is a bit late compared to other fields (e.g. Computer Science which has taken data mining and machine learning, Operations Research [INFORMS] which has taken Business Analytics), it is better late than none.
That said, the article focused on the technical skills but missed the other important skills to be a good “data scientist” (other similar terms: data miner, decision scientist, business analyst). To be a good “data scientist” or a good applied statistician, one must have great communication skills, be a strong team player (collaborating with others from various fields), and must have some subject matter knowledge – to mine marketing data, one must have some basic marketing and economics knowledge; to analyze genomic data, one must have some basic understanding of genomics; to handle risk analytics, one must know something about risk management, economics, and finance, etc.. Without having the subject matter knowledge, it could be dangerous in your analysis and it would be very hard to communicate with colleagues (as a statistician, it’s our job to translate our terminology to theirs, do not expect the other way around). Unfortunately, most formal degrees in statistics do not teach these key skills, so one would need to acquire it in other ways. Some schools have seen this need and have introduced analytics, business analytics, or predictive analytics degrees that cover not just the technical skills but these other skills as well. Stat departments have a choice: stick with the current way or adapt.
Toby,
Word clouds would only be the silver bullet you suggest they are if the relationship between word and topic were 1:1. Unfortunately, that’s not the case.
The challenges of big data and data mining are that the data is difficult to analyze, difficult to represent appropriately, and difficult to place in terms of ‘what it means.’
I agree with Anon, that it is great to see ASA talking about Big Data. Our field is at the precipice of a big change, and we need to make sure that the change happens with a high degree of rigor and data integrity. I hope that ASA will play a role in that.
Welcome!
Amstat News is the monthly membership magazine of the American Statistical Association, bringing you news and notices of the ASA, its chapters, its sections, and its members. Other departments in the magazine include announcements and news of upcoming meetings, continuing education courses, and statistics awards.
ASA HOME
Departments
Archives
ADVERTISERS
PROFESSIONAL OPPORTUNITIES
FDA
US Census Bureau
SOFTWARE
STATA
QUOTABLE
“ Looking back, I can say with certainty the ASA has been an integral part
of my professional journey.”
— Weijie Su, Associate Professor
Editorial Staff
Managing Editor
Megan Murphy
Graphic Designers / Production Coordinators
Olivia Brown
Meg Ruyle
Communications Strategist
Val Nirala
Advertising Manager
Christina Bonner
Contributing Staff Members
Naomi Frieman, Elizabeth Henry, Kim Gilliam
Contact us
Amstat News
American Statistical Association
732 North Washington Street
Alexandria, VA 22314-1904
(703) 684-1221
www.amstat.org
Address Changes
Amstat News Advertising