Statistics, AI, and Autonomous Vehicles
David Banks and Yen-Chun Liu
Yogi Berra said, “It’s hard to make predictions, especially about the future.” But making quantified predictions is something statisticians are supposed to do pretty well.
In this case, we want to examine the future of statistics in artificial intelligence for autonomous vehicles. The exact service statisticians eventually provide will depend on the interplay of technology, law, economics, and social adoption of AVs. We shall be called upon to do risk analyses, probably under many scenarios (e.g., for a mixed fleet and in bad weather). We shall make estimates of the impact of AVs on the environment and economy. We are likely to play a role in insurance and regulation. We may be asked to develop procedures to assess the quality of AI software and the completeness of AI training.
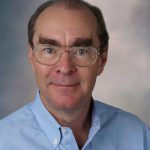
David Banks earned his master’s in applied mathematics from Virginia Tech, followed by a PhD in statistics. He won an NSF postdoctoral research fellowship in the mathematical sciences, which he took at the University of California, Berkeley. In 1986, he was a visiting assistant lecturer at the University of Cambridge, and then he joined the department of statistics at Carnegie Mellon in 1987. Ten years later, he went to the National Institute of Standards and Technology, then served as chief statistician of the US Department of Transportation, and finally joined the US Food and Drug Administration in 2002. In 2003, he returned to academics at Duke University.
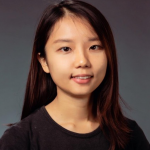
Yen-Chun Liu is a second-year PhD student in statistics at Duke University with research interests in surrogate modeling, Bayesian optimization, causal inference, and reinforcement learning. Before joining Duke, she earned her bachelor’s degree in mathematics at National Taiwan University and master’s degree in statistics at National Tsing Hua University, Taiwan. Besides studying statistics, she enjoys engaging in outdoor activities and reading. Liu is also passionate about various social issues, including environmental sustainability and social justice.
AVs could be transformational. They can significantly reduce pollution, save lives, and provide other benefits. They are one of the few technologies with the potential to meaningfully mitigate climate change.
The greatest benefits would accrue if all vehicles on the road were networked AVs. There would be little need to brake, which is wasteful of fuel—cars could adjust speeds to interweave without stopping and restarting at stoplights. Platooning would also save fuel. And if AVs become sufficiently safe, we no longer need to carry around 1.5 tons of steel for protection. Car bodies could be made of canvas, saving yet more fuel. In terms of safety, AVs are never tired or distracted, and they have better sensors than human-piloted vehicles. If networked, the AVs can share information about safety conditions such as a herd of deer near a road.
According to the National Highway Transportation Safety Administration, there were 42,939 crash fatalities in 2021. The National Safety Council reports that about 20,000 involved multi-car collisions. If only networked AVs were on the road, this number would presumably drop to nearly zero.
Among the 9,026 single-vehicle fatal crashes in 2021, 59 percent of drivers had blood alcohol levels above 0.08. Not all these fatalities were due to alcohol use, but NHTSA has much evidence to suggest alcohol is a dominant factor in vehicle deaths. Networked AVs would also eliminate deaths and injuries caused by inexperienced teen drivers and elderly drivers with diminished capability.
Modern work using software to pilot robotic vehicles began in 1984, when William “Red” Whittaker launched the NavLab project. In 1995, NavLab 5—a small truck carrying a computer—drove from Pittsburgh to San Diego, mostly without human guidance. Another roboticist involved with that team at Carnegie Mellon University was Sebastian Thrun, who played a leading role in Google’s initial effort to build AVs, which has now been spun off as Waymo.
Following are the six levels of vehicle automation:
Level 0. The human has only standard assistance (mirrors, rear-view cameras).
Level 1. Software assistance. Examples include adaptive cruise control and lane keep assist. This level became widely available after 2018.
Level 2. Partial automation. The driver must be hands-on and ready to take control, but the car controls speed and holds its lane. The Tesla Autopilot is an example.
Level 3. Conditional automation. Hands are off the wheel, but the driver must still be ready to control. It is intended for limited access highways and good driving conditions. Many automobile companies are experimenting with this level.
Level 4. High automation. Driver can sleep after inputting destination. Waymo is testing these kind of AVs. The car must stay on traditional roads.
Level 5. Full automation that enables navigation of nontraditional roads.
From a statistical risk analysis standpoint, we are beginning to acquire significant data on Levels 3 and 4.
Waymo is essentially the only company operating Level 4 vehicles. They report that, as of January 2023, they had driven a million AV miles. The news release states there have been no injuries and 18 minor contact events. Of those, 10 involved a human driver hitting a stationary Waymo AV. It asserts the human operator violated road rules in every vehicle-to-vehicle collision.
It is difficult to compare the Waymo record to human performance. The number of miles driven is too small to compare fatalities and injuries are difficult to define. Nonetheless, the Bureau of Transportation Statistics reports there were 2,250,000 roadway injuries and people in the US drove a total of 2.9 trillion miles in 2020.
One would have expected 0.78 Waymo injuries if Level 4 AVs are identical to human drivers, but there was none. There is insufficient evidence to conclude Level 4 vehicles are safer than humans, but it strongly suggests Level 4 AVs are not worse. Note that Waymo operates mostly in the metropolitan areas of Phoenix, Arizona, and San Francisco, California, and injury rates tend to be higher in urban areas.
Obviously, statisticians in the BTS and NHTSA are better placed than we are to do risk analyses that control for driving conditions and the kind of accidents that occur. This is clearly an important role for statisticians, and AV safety should be monitored.
Since July of 2021, NHTSA has required AV manufacturers to report crash data. As of January 15, 2023, NHTSA says carmakers have submitted 419 AV crash reports. Of these, 263 have involved Level 2 vehicles, with 156 involving Level 3 or higher AVs. NHTSA further reports 18 fatalities, all with Level 2 cars. No carmaker has reported a fatality with a Level 3 or higher AV, but it should be noted there are far fewer of these on the road. The California DMV reports Level 3 AVs drove a total of 10.4 million miles between January 2021 and December 2022, but that is too small to warrant a risk assessment, since the fatality rate per 100 million miles driven is only 1.34 in 2020.
The NHTSA report has 19 accidents for which the injury level is listed as “unknown.” Also, NHTSA does not capture whether the AV was at fault in the crash, nor whether the accident was caused by user error or a problem with the AI. Nonetheless, statisticians at NHTSA are uniquely situated to undertake risk analyses that flag common failure modes.
All current risk analyses pertain to the mixed fleet situation, but the greatest safety benefits accrue when all vehicles on the road are networked AVs.
Scenarios
There are several ways AV use might develop. One is the mixed fleet situation we have now. The data hints that, on average, AVs are somewhat safer than humans. This scenario will evolve as car manufacturers and statisticians learn more about how specific levels of AV perform. We can imagine that one day a person might unlock their Level 2 AV and the car will say, “You will have to drive yourself today. There is snow on the road and the AI feels it is not able to operate the automobile safely.”
This scenario will lead to new regulations, legal decisions, and insurance policies. The regulations would probably prescribe how software updates should be made securely in a world where international cyberattacks have become common. Similarly, they would govern inspection frequency, the precise capability of different levels of AV, where AVs could be driven, and so forth. Legal decisions would determine liability in crashes, which in turn would drive new forms of insurance policies.
A second scenario, not incompatible with the first, is that a push for AV adoption will come from the trucking and/or ride-share industries. Both will profit if driving can be automated (with additional benefits from all-electric fleets). Perhaps one lane of the interstate highway system will become dedicated to AV trucks. When an AV truck leaves the highway, a human operator might take control, as Air Force pilots direct international drones from domestic bases. Similarly, Waymo is operating a ride-hailing system in Phoenix and San Francisco. Change may happen in tandem; Uber is building a freight fleet that uses Waymo’s self-driving trucks.
A third aspect of an AV future is that many vehicles will probably look different than transportation built to move humans. During the pandemic, there was an increase in online purchases, which led to deliveries that encountered the “last mile” problem. One can efficiently move goods from the site of manufacture to the depots near purchasers, but then panel trucks are needed for delivery. These panel trucks might be replaced by AVs that look like an electrically powered grocery store shopping cart.
It is entirely possible that the world will move away from the concept of car ownership. Instead, people might gravitate toward a ride-hailing system. A family of six could summon a large van when traveling together, a fuel-efficient single-seater when only one person is going, or a luxury vehicle when traveling a long distance. Additionally, a ride-hailing system lends itself better to the use of electric vehicles since the AV can take itself off the road to recharge.
Training an AV
All AVs use deep neural networks. Training a deep neural network requires lots of data, an appropriate architecture, and an optimization algorithm. The architecture is often modular, with subnetworks trained to perform specific recognition or prediction tasks. The optimization algorithms are proprietary but probably quite complex and specific to given subproblems. A limiting factor in training neural networks is they need human beings to categorize unrecognized images such as a heavily weathered stop sign.
There are constraints. Latency must be short so the AI can react quickly to changing traffic conditions. Neural network code must be simple enough to store onboard. The implementations evolve over time and are highly proprietary. Statistical thinking arises in the training of deep neural networks, but it is not central, and so our review of this aspect of AVs is brief.
Initially, the goal was to have an AV that held its lane and followed a car in front at a fixed distance. But now, more complex behavior is wanted. In the Tesla AV, there are multiple camera sensors whose raw images are processed by a rectification layer in the deep network to correct for miscalibrated cameras. These images are sent to a residual network that processes them into features at different channels and scales—features might correspond to stop signs, lane markings, and other vehicles. Features are fused into multiscale information by a module that represents it in a vector space. The output space is sent to a spatio-temporal queue processed by a recurrent neural network. The output is fed to a component called the hydranet, which sends the processed data to modules that handle different prediction tasks. Inez Van Laer provides an outline of the algorithm in “Tesla’s Self-Driving Algorithm Explained,” which also includes a link to a YouTube video on the topic by Andrej Karpathy, Tesla’s director of AI and autopilot vision.
Training an AV AI can be gamed. Applying a Post-it note to a stop sign can fool an AI into thinking the stop sign is a billboard.
Regarding training, it is worth noting that Tesla has driven far more miles than Waymo or any other AV system. As of July of 2022, it had driven 35 million miles. In 2019, it had a fleet of around 5,000 vehicles and drove as much in a day as Waymo has driven to date. When a Tesla AI encounters a new situation, or ‘sees’ something it doesn’t recognize, it records it to add to the training database. This means it has more data, thus the potential to jump from Level 3 to Level 4.
Impact
AVs will have a major economic impact. They lower the cost of production (e.g., less expensive supply lines, fewer distribution costs). There is more fuel efficiency, and drivers are not paid to pilot trucks. Such change would cause significant dislocation in employment, and an ethical society should plan how to ameliorate the impact on people who lose jobs for the benefit of all.
AVs will have a significant social impact. We may shift from personal cars to a ride-hailing system. Children could be delivered to school by an AV. The elderly could stay in their homes after no longer being able to drive. Before COVID-19, the US Census Bureau estimated the average time spent commuting was 55.2 minutes per day. AVs would allow people to read, work, or sleep, thus giving them an extra hour each day.
But the economic and social impact of AVs is dwarfed by their potential environmental impact. The world’s population is increasing. According to the Census Bureau, there are 7.97 billion people now and there will be 9.5–10 billion by 2050.
Researchers at the Australian Academy of Science say the carrying capacity of the planet with anything like a middle-class American lifestyle is on the order of 2 billion. There is no technology on the horizon that can convert a ton of sand into a ton of food and use little energy to do it. But that is the scale of the problems we face.
Climate forecasting is less accurate than demography, but scientists at NASA estimate parts of South Asia, the Persian Gulf, and the Red Sea will be too hot to support human habitation by 2050. And by 2070, the same will be true for parts of Brazil, eastern China, and southeast Asia. Geopolitically, there will be consequences. The people of Bangladesh (who also face problems from the rising sea level) will have to move north to Pakistan or Afghanistan—a relocation fraught with peril.
Similarly, the political governance in Syria and Lebanon is precarious. Climate pressure—say a week of 120-degree temperatures—could lead to collapse and further waves of refugees. The only way for people in such regions to survive is through access to potable water and technology. Many nations in those regions do not have such capacity now and may not acquire the resources in time.
No government in the world has the political will to leave coal in the ground unburned when it is 120 degrees outside and its people need to run a desalinization plant. That sets up a vicious cycle in which more carbon is produced, causing higher temperatures, which leads to more carbon being released.
Which brings us back to AVs. They are one of the few technologies that could have a meaningful impact on our future’s carbon footprint. The US Environmental Protection Agency says transportation accounts for 29 percent of US greenhouse gas emissions; it is the largest single component of our pollution budget. In the United States, transportation accounts for 41.7 percent of CO2 emissions (77 percent if one excludes wildfires). Networked, electric AVs could dramatically mitigate global warming while simultaneously improving our quality of life socially and economically.
AVs have enormous potential impact, and forward planning can achieve important social and economic benefits, as well as essential environmental benefits. AVs depend upon AIs, which are trained upon vast quantities of data. Statistical methods are key to many aspects of AV implementation. Risk analysis is obvious, but our community can and should play a much larger role.
Editor’s Note: View an academic version of this paper, complete with references showing heavy use of work by statisticians from several government agencies.
How do you fix an AI vehicle?
Pull over to the side of the road. Pull out your handkerchief … and wipe the bug splatter off the car’s front sensors.
Based on an Arizona highway experience with a self-driving car.
Leave your response!