How Can Statisticians Contribute to the Evaluation of Foundation Models?
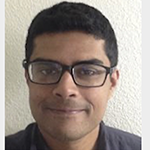
Giri Gopalan recently joined the statistical sciences group at Los Alamos National Laboratory as a staff scientist. Prior to this, he was assistant professor at California Polytechnic State University, San Luis Obispo and visiting assistant professor at the University of California, Santa Barbara, both in statistics.
Natalie Klein is a staff scientist in the statistical sciences group at Los Alamos National Laboratory. She holds a joint PhD in statistics and machine learning from Carnegie Mellon University.
Emily Casleton is the deputy group leader of the statistical sciences group at Los Alamos National Laboratory. She joined the lab as a post doc in 2014 after earning her PhD in statistics from Iowa State University. Since converting to staff in 2015, Casleton has routinely collaborated with seismologists, nuclear engineers, physicists, geologists, chemists, and computer scientists on a wide variety of data-driven projects.
IJ Good, a prominent mathematician and Bayesian statistician who worked alongside Alan Turing at Bletchley Park during World War II, anticipated the rise of ultra-intelligent machines in his 1966 “Speculations Concerning the First Ultraintelligent Machine.” In concluding remarks, he wrote the following:
Good’s statement appears to be rather prescient. While humanity has marched on through to the 21st century, modern artificial intelligence—driven by a menagerie of immense deep-neural-network architectures aided with parallel computation—seems positioned to change life as we know it. The world has been captivated by AI. Recent engineering feats such as autonomous vehicles and generative AI have gripped the public sphere, and AI is poised to disrupt a host of industries, including software, pharmaceuticals, health care, manufacturing, and entertainment. Indeed, one is hard pressed to find any industry that does not claim to bear an impact from the so-called AI revolution.
Possible candidates for or precursors to Good’s ultra-intelligent machines are foundation models, a class of models that includes popular contributions such as ChatGPT, Gato, Stable Diffusion, and LLaMA. Such a grandiose term might conjure the Standard Model of particle physics. But in the present context, foundation models can be thought of as prodigious machine learning models—often deep neural networks—that have been trained on a large aggregate of data and adapted to solve a variety of downstream tasks. In this sense, foundation models are foundational for specific downstream problems. This is in contrast to the once standard approach of building entire specialized machine learning models from scratch for each task. For instance, a large language model might be trained by giving it a large corpus of sentences and asking it to fill in missing words that have been randomly removed. The resulting model can be fine-tuned to solve related language tasks such as sentiment analysis. Thus, with just a single foundation model, one can efficiently adapt to solving a large set of complex tasks, which is in line with the concept of an ultraintelligent machine articulated by Good in 1966.
A statistician might wonder what they can contribute to the game. Is the profession obsolete given the advent of AI and foundation models? Quite to the contrary. Following are a few key ways basic statistics knowledge can contribute to the evaluation and comparison of foundation models:
1. Quantifying uncertainty associated with scores: It is common to rank machine learning model performance based on point estimates of a score (e.g., accuracy) evaluated on a benchmark data set. This approach inherently ignores uncertainty in the metric (e.g., due to sampling variability) that could be quantified with a standard error. Basic normal theory confidence intervals can be used to estimate standard errors when asymptotic arguments apply; when such asymptotics are not plausible, one might use resampling techniques such as the bootstrap or Bayesian methods. Nonetheless, whether or not a credible or confidence interval is used, some defensible assessment of uncertainty is preferred to none when comparing machine learning models, and many foundation model leaderboards currently ignore uncertainties in their rankings.
2. Aggregating performances across tasks when comparing foundation models: A new challenge specific to comparing foundation models is how to best aggregate their performances on a set of tasks. Typically, existing foundation model benchmark leaderboards rank competing foundation models by using unweighted averages across scores on different tasks. A more nuanced approach would take into account variance on score estimates across tasks. For instance, scores on difficult tasks with more questions should be weighted more heavily than task scores with fewer, easier questions. Additionally, care must be taken to normalize scores before combining them. For example, how should one combine an accuracy score (i.e., between 0 and 1) on a classification task with a mean square error (non-negative but unbounded) on a continuous prediction task? Statistics ought to be able to provide normative answers to such basic questions.
3. Quantifying uncertainties on predictions of foundation models: An important aim is to quantify uncertainty associated with predictions derived from foundation models. Because foundation models are usually too large to iteratively refit, one must obtain predictive intervals that are inherently conditional on the fit foundation model—for which post-hoc calibration, conformal prediction, and approximate Bayesian methods like Monte Carlo dropout and Laplace approximations may be appropriate. Predictions with uncertainties can help consumers gauge how much to trust the output of foundation models, and they can help inform experimental design strategies for gathering a test set (e.g., with sequential design as opposed to oft-assumed IID sampling methods).
These are but a small fraction of ways statisticians can contribute substantively to AI without offering new varieties of AI models. Statisticians have an important role to play in the landscape of rigorous AI deployment, especially with regard to the evaluation of foundation models, AI, and machine learning more broadly.
Leave your response!